On the 22nd of this month, Stability.ai released Stable Diffusion, a free, open-source AI tool for generating images from text prompts (and other images). Previously, access to this technology was available through Open AI’s commercial, invite-only DALL-E 2 platform, Midjourney’s commercial Discord bot, and, in a significantly reduced free-to-use form, Craiyon/DALL-E Mini. Basically, you had to use a hosted service and pay per image, or use something too simple to be much use for anything more than amusing jokes to post on social media.
Stable Diffusion’s public release is a massive sea change. Now, anyone with a reasonably powerful computer can use this tech locally to generate strange and fantastical images until their graphics cards burn out. While DALLE-2 is still probably the more powerful system, able to generate better results from shorter prompts, outputs from Stable Diffusion can rival those of its commercial competitors with a bit of careful tweaking – tweaking made possible by users’ increased control over its parameters.
For comparison, two images made with the same prompt:
the city of cape town as a martian colony artist's conception landscape painting
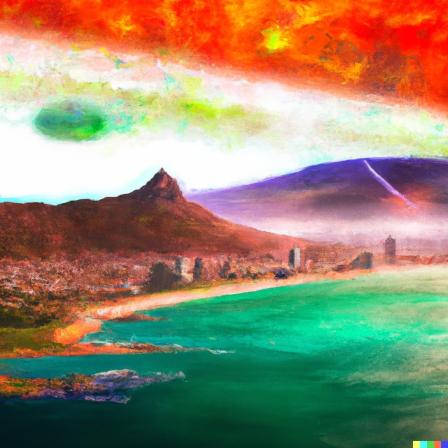
DALL-E 2
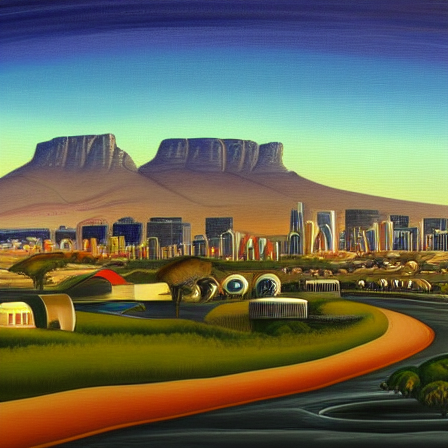
Stable Diffusion (Euler Ancestral sampler)
DALL-E’s is more lush and naturalistic, whereas Stable Diffusion produced something very precise and, well, clearly computer generated, though not without its charm. Moreover, the other three images DALL-E generated for this prompt are just as good, whereas I had to fiddle with different samplers and iteration counts to get something I liked as well from Stable Diffusion. But, crucially, making extra iterations didn’t cost me any credits.
Stable Diffusion really shines when you give it a style to imitate. This can be an artist’s name, an animation studio, or something like “comic book inks”. The best results often combine multiple artist names and styles. I quite liked what I got from having Flemish Renaissance painter Joachim Patinir redo my Martian Cape Town.
city of cape town, mars, martian colony, artist's conception, highly detailed, 8k, intricate, artstation, landscape, by Joachim Patinir
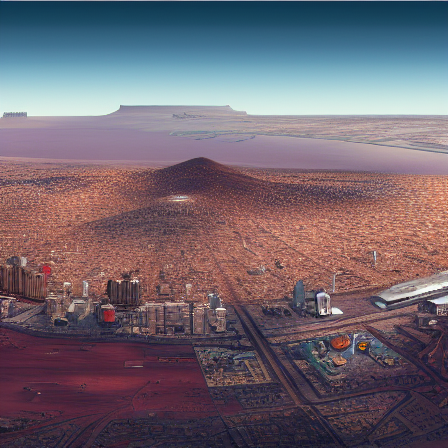
Stable Diffusion (Euler Ancestral sampler)
In addition to specifying a prompt, Stable Diffusion allows you to tweak the following parameters:
- Seed
- The random seed used to initialise the generation. By controlling the seed, you can create reproducible generations, which is key to experimenting with prompt and configuration variations – using slightly different prompts with the same seed gives you some idea of what part of the image each word is responsible for. This is exactly the kind of technical detail that DALL-E’s slick, user-friendly interface doesn’t let you touch.
- Sampler
- The k-diffusion sampler used to generate each iteration of the image from the last one. /u/muerilla provides a useful illustration of this on /r/StableDiffusion:
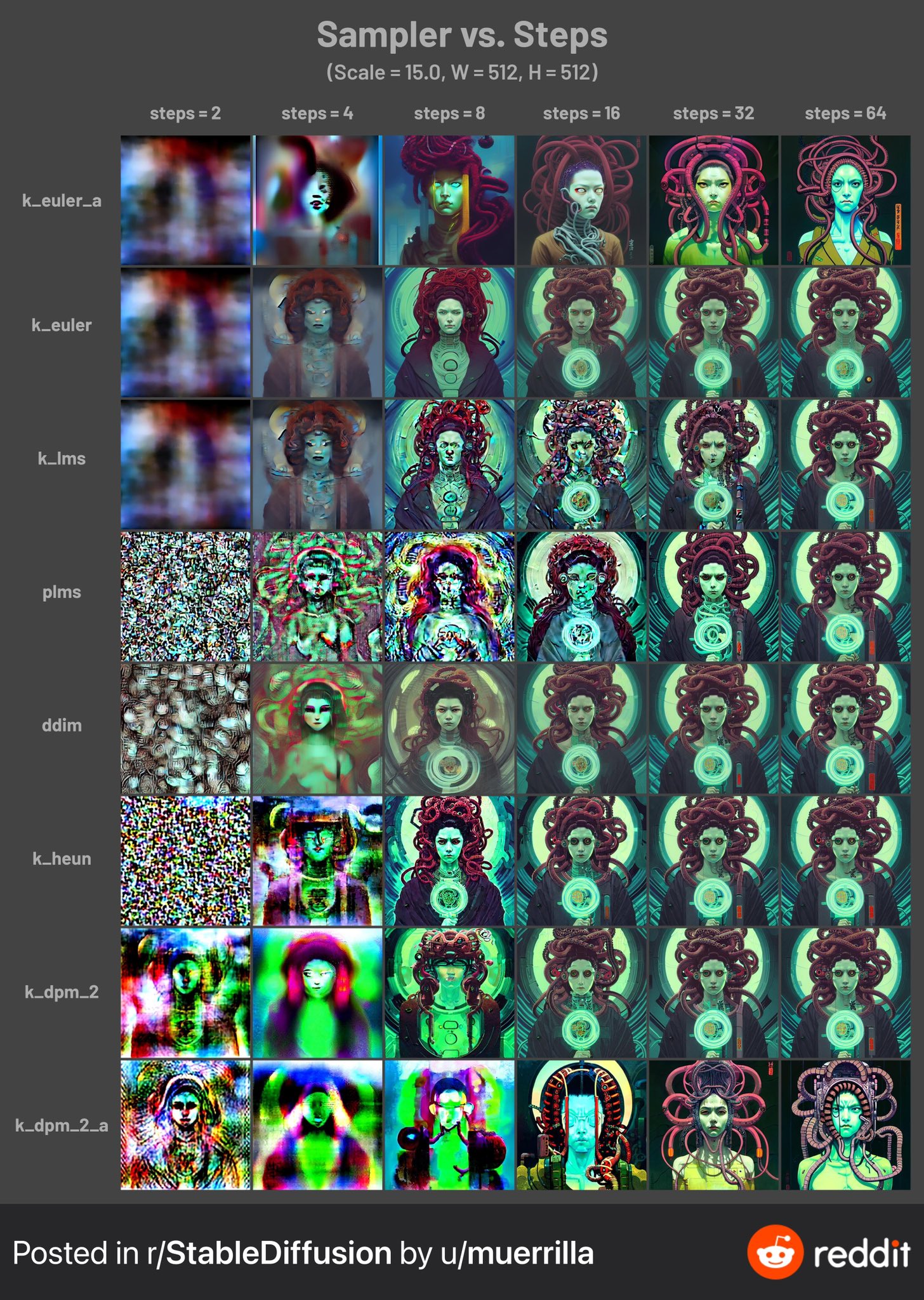
I use k_euler_a
most often because it produces the quickest results.
- Steps
- The number of sampling steps to take, also illustrated by the figure above. Initially, additional steps will lead to a more coherent and detailed image, but this reaches a point of diminishing returns somewhere in the hundreds of steps.
- CFG scale
- Also called the unconditional guidance scale, this is a number specifying how closely you would like your output to align to your prompt. The default value is 7.5. Lower numbers allow Stable Diffusion more artistic licence with the output, generally resulting in trippier images. Higher numbers ensure you’ll get what you asked for, but the results sometimes look crude or overcooked, reducing Stable Diffusion from a skilled artist to a Photoshop beginner. Generally, higher CFG scales work better with more detailed prompts and higher step counts. The images below have the same seed and prompt, but different CFG scales:
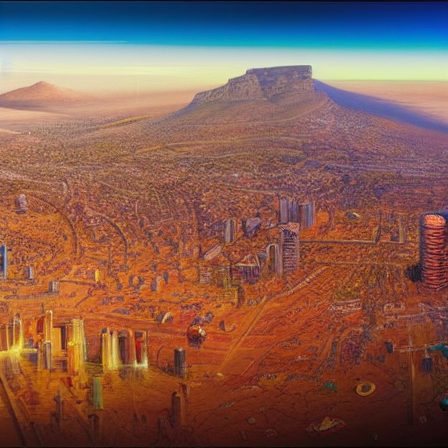
CFG 5
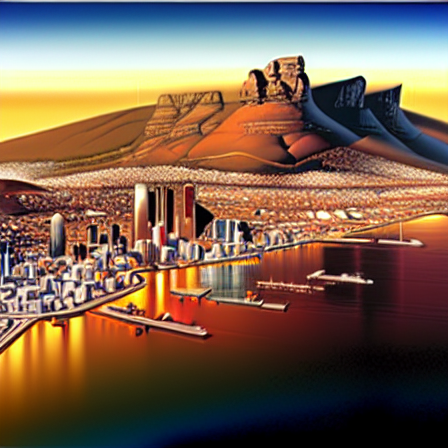
CFG 30
- Dimensions
- Image dimensions will significantly affect your output. Stable Diffusion was trained on 512*512 resolution images, so this is the recommended setting. Anything bigger than 1024*1024 is not officially supported and can cause tiling, and anything smaller than 384*384 will be too messy and artifacted to come out looking like anything. Also, high output resolutions require powerful graphics cards. My system, on the lowest end of the supported hardware, can’t handle anything bigger than 448*448.
Below, I’ve generated three images with the same seed, prompt and other configuration values, but different dimensions:
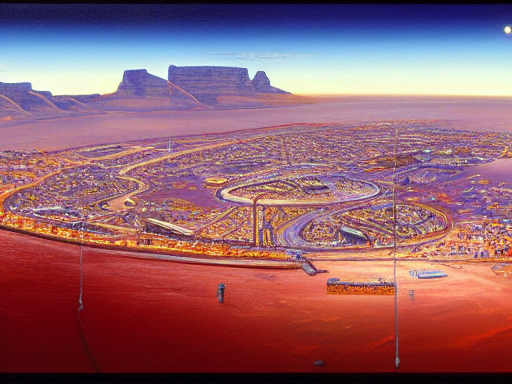
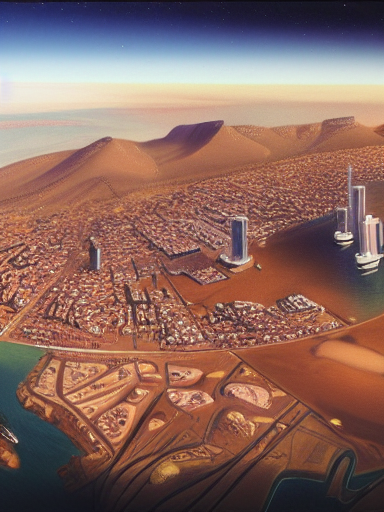
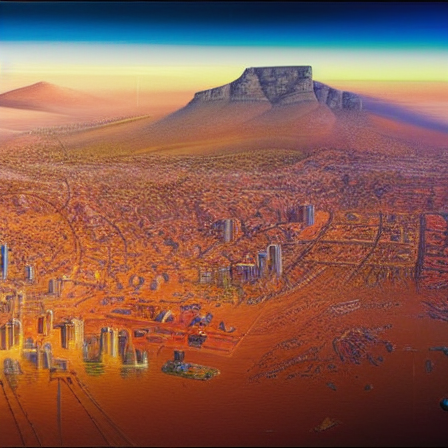
Stable Diffusion can generate more than just landscape paintings. It’s also pretty good at portraits, with enough of the right keywords.
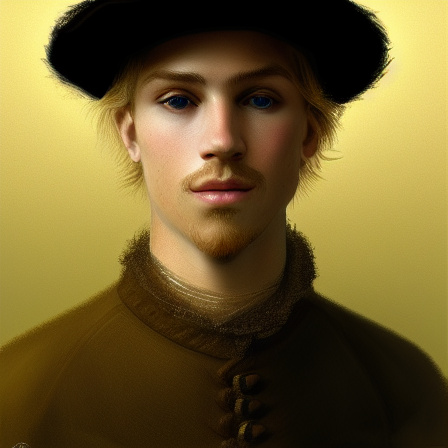
Portrait of a young man
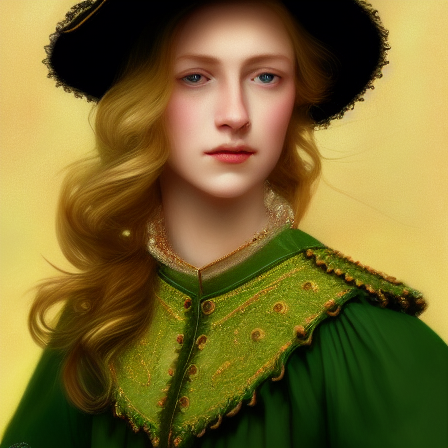
Portrait of a young woman
Above, I’ve used the same seed with slightly different prompts to generate portraits in the same style of two people who could be siblings. DALL-E 2 and Midjourney both let you generate variations of images you like, but what’s actually varied is a bit random. The ability to tweak a prompt for the same seed lets you have much more control over the direction a variant goes in. We can even get (roughly) the same character at different ages:
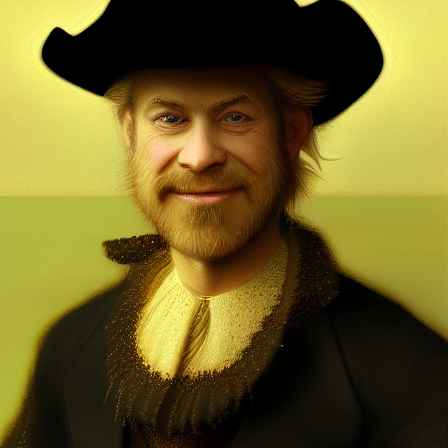
Portrait of an older man
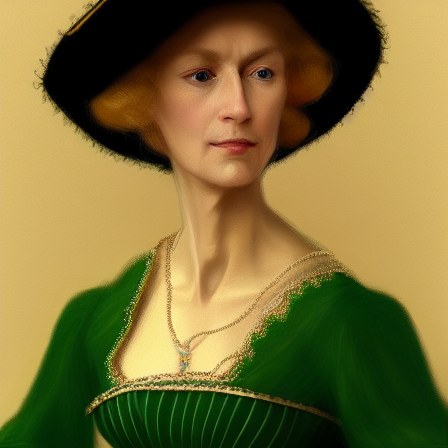
Portrait of an older woman
Things get a little dicier once you move from portraits to full bodies. Stable Diffusion is prone to generate extra arms and legs at lower CFG scales, and even otherwise perfect pictures usually come with deformed hands and feet.
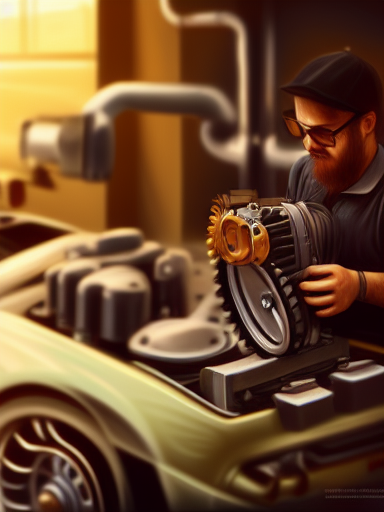
Unusually coherent hand, warped engine part
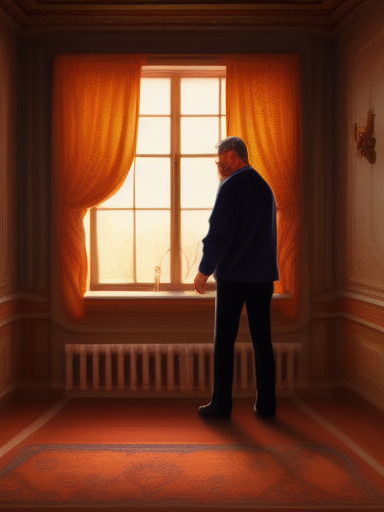
Face and hands are small enough that imperfections aren’t that noticeable
Trying to get anything in motion – characters walking, eating, etc – often leads to horrific nightmare images not unlike the ones generated by Craiyon, but in ghastly high resolution. This is where Stable Diffusion’s other tool, the image to image generator, comes in. It’s a variation on the text to image generator that lets you specify an original input image to work with, in addition to a prompt. This can literally be a crude MS Paint drawing. I made a steampunk robot:
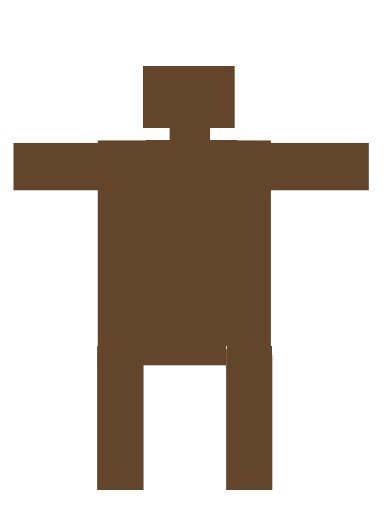
1 million hours in Pinta
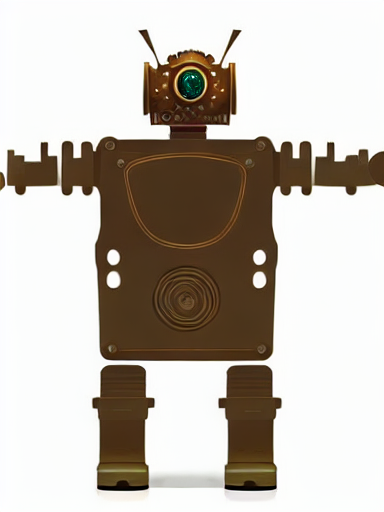
image2image, single run
Digital artists are using this with sketches to great effect.
But you don’t just have to start with your own drawing. You can start with a photo, and convert it into a painting, or vice versa. You can also take output from text to image, draw some coloured blobs on the parts you want to change, and get image to image to redraw it.
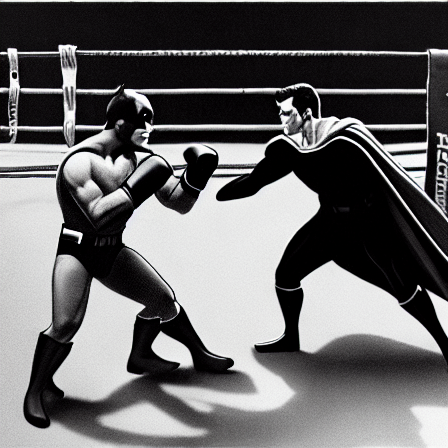
Text to image generation
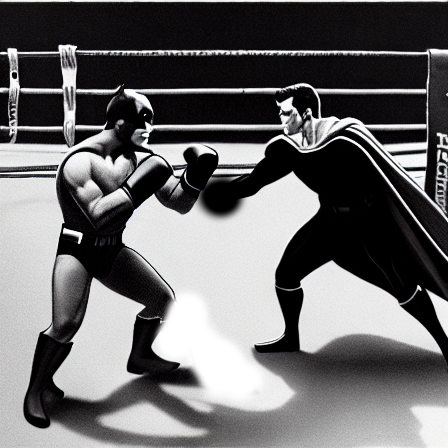
Manual addition of hand & removal of extra leg
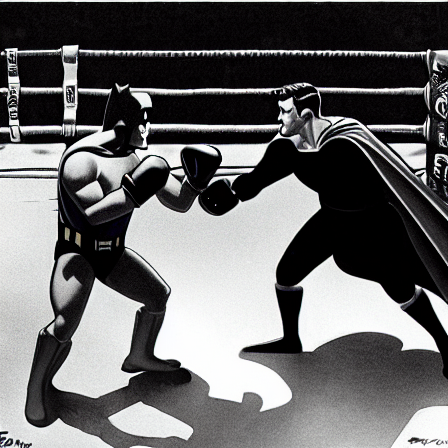
Image to image generation
Note how the final image even fixes the Batman’s double right foot on its own. We would probably need to go through a few more iterations to get the faces looking decent. For example, we could generate the faces on their own, shrink and paste them in, and then use image to image produce a blended result. For more targeted fixes, some Stable Diffusion GUIs have built-in masking interfaces, where you can select one single part of an image to regenerate, avoiding the small changes you’re bound to get on multiple runs over the whole thing. And we can also use other AI tools like GFPGAN and ESRGAN to fix faces and increase resolution.
Writing prompts for the AI is a bit like learning a new language. A lot of the words you can use in prompts are straightforward and logical – “portrait of a man” will come out as you expect. But as you get into more complex territory, you have to learn the peculiar meaning of terms like “trending on artstation” and try to find ways to ask for different angles without getting a lot of cameras and fish eyes in your results. You can start to figure it out by doing a lot of trial and error with the same seed, adding and removing terms; by looking at what others have generated; by searching the training dataset; or even by generating prompts from images. And if none of that works, you can buy prompts other people have figured out.
Amusingly, terms like “masterpiece” and “award winning” also help to produce attractive outputs. “Just draw an award-winning masterpiece for me, would you?”
It’s amazing how far this technology has come from dog face mosaics. And it’s still developing at a break-neck pace. I mentioned using seeds and prompt variations to generate variations of the same character above, but there’s a much more robust way to do this called textual inversion, which you can test out now if you have a very beefy graphics card. Hopefully, DALL-E-style outpainting will also come to Stable Diffusion.
The question of copyright is a fraught one. Fundamentally, these AIs work by taking in a huge volume of images, most of them copyrighted, and mushing them together in a black box that no-one really understands. You could argue that this is similar to how a human artist might learn things, but these AIs far outstrip the ability of any human across many metrics. Fundamentally, they’re inhuman. And when you can get them to generate images drawn in the styles of living artists, well, not all of them are going to be receptive to that. Simon Willison brings up the point that AIs could be trained on public domain works only, but whether you as an individual are going to use the ethically sourced AI or the standard one is going to be a personal choice.
I’ve personally been much more interested in using Stable Diffusion than I ever was in text generation systems like GPT-3 and AI Dungeon. I’m a far better writer than artist, so the idea of generating text from a prompt is an amusing diversion at best. If I want some text, I’ll just write it myself. Although some artists are already integrating AI into their workflows, others will probably have the same meh reaction to its applications in their field.
Whatever happens now, this toothpaste is not going back in the tube. The cloud continues to correlate its contents.